Factories and plant floors are generating unprecedented volumes of data today. The proliferation of IoT sensors is enabling companies to extract insights from these disparate data sources. However, as a startup aptly described, industrial customers suffer from the ‘data rich, information poor’ syndrome. Customers underutilize data because of a lack of sophisticated analytics tools. Consequently, the industrial IoT (IIoT) analytics market is increasingly emerging as the hotbed for startups.
After spending considerable time interacting with startups, companies, sales and engineering partners in this space, I’d like to cover three topics in this blog series:
Fundamental data challenges specific to IIoT
The current state of the analytics ecosystem
Key go-to-market (GTM) and business model strategies for startups to win in the IIoT analytics market
In this blog, I refer to IIoT analytics as platforms that provide predictive insights on assets, machines and industrial processes. Under Operational Technology (OT), I refer to verticals such as manufacturing, automotive, chemicals, mining and metals, energy and utilities.
In terms of end outcomes, OT customers care about a single-pane-of-glass visual tool on their site data. A Tableau-like interface can significantly improve their productivity and asset optimization.
Analytics use cases fall into two main categories:
Install Base Analysis: Operational visualization of site data. Examples include machine location, types of machines, uptime, downtime, capacity utilization, bottleneck and root cause analysis. Typically, visualization is the first table stakes offering and becomes the anchor point to upsell predictive analytics.
Predictive Maintenance: Anomaly detection and alerts, if machines behave unexpectedly.
OT managers lack even a complete step 1 – they don’t have a full picture of what their asset inventory is. Such analysis is largely done offline and manually through Excel / CSV files today. Most industrial customers are still in a data acquisition phase. They are not ready for the purported AI revolution – they simply want to extract data from disparate machines.
I am excited by this market and believe there is a massive opportunity for startups to revolutionize the industrial world and capture a significant chunk of the industrial wallet. In order for that day to arrive however, startups need to work through some data and GTM challenges to reach their potential.
OT-specific data challenges
At the highest level, the typical technology stack and flow of IIoT data looks like this:
Through the journey from the sensor to the visualization dashboard, there are fundamental challenges specific to OT data. Simply put, data is difficult to collect, ingest and train for analytics.
Startups need to work through the following data challenges, as they build their platforms:
Data Collection – Too many protocols
Data interoperability and integration are complex in the industrial world. Brownfield industrial machines and control systems speak in legacy, proprietary machine-to-machine protocols. Historically, standard protocols were never built for these devices, which have existed for 15 – 20 years in customer sites. Vendors often need to build custom protocol connectors to heterogeneous sources and convert the data into standard protocols. The process is fairly manual because the protocols can be manufacturer- and device-specific. As someone simply explained to me, you need to convert the sensor-generated data from German to English, so that analytics engines can understand the data.
Data Management – Incompleteness of data
Poor connectivity in industrial environments leads to data integrity issues. Sensor-generated time stamps (humidity, pressure metrics etc.) are often lost or inconsistently recorded. To properly train the analytics models, data preparation and enrichment is manual and cumbersome.
Predictive Analytics – Data modeling issues
Sensor data is usually stored in time-series databases called historians. Pure time series data inherently lacks context. While there are standard modeling techniques, domain-specific knowledge is critical in order to contextualize machine interactions. Startups often lack the resident OT knowledge to define the right time intervals and actionable variables, map the varied machine relationships and reduce false positives. Consequently, startups need big application development teams to work with OT customers to build the right data models, which are very specific to industrial settings.
In this regard, OT data challenges make it difficult for startups to build a pure self-service product. They need to constantly provide professional services to build integrations and custom-applications.
___________
IIoT Analytics Landscape
The market is fragmented and early, with most customers in pilot phases. For startups, the good news is that the bigger companies have not cracked the market yet and there is a massive opportunity to fill this whitespace. Bigger OT ecosystem players are hungry to work with analytics startups, in order to provide stronger bundled solutions to customers.
Here is the current landscape of the big ecosystem players that have IIoT analytics products:
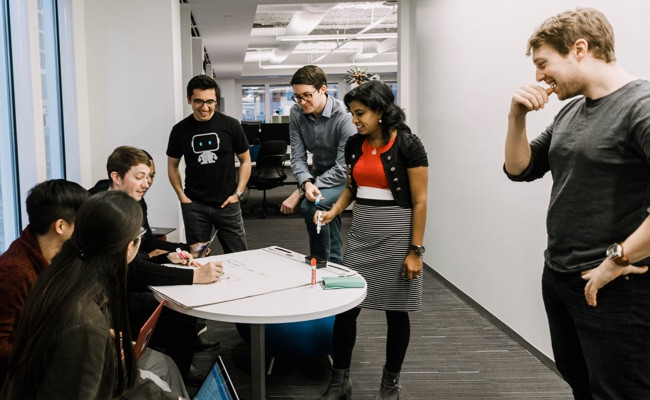
Note: The companies listed are some of the major vendors in their respective categories. This is not an exhaustive list or an endorsement.
Device makers:
Industrial automation manufacturers such as GE, ABB, Siemens and Schneider, have taken a bottom-up approach – they upsell their industrial equipment with data platforms.
These platforms have seen mixed results in the market (GE Predix in particular). Historically, industrial manufacturers have built machine control software, but lack the machine learning expertise for predictive analytics.
It is also unclear how they can evolve horizontally outside of their individual OEM ecosystems. Customers operate multi-vendor sites and platforms from GE, Siemens, often fail to provide broad analytics on machines from different manufacturers. These platforms can often lose out to more neutral, horizontal vendors.
Going forward, I predict that industrial manufacturers will be the most acquisitive in the space. In the commoditized industrial machinery market, analytics is a big differentiator, and increases the upsell potential for hardware and services pull-through.
OT infrastructure companies:
The four main cohorts include edge analytics, middleware, security and networking. Vendors in this space only offer piecemeal analytics solutions, mainly related to networking and data ingestion.
With massive amounts of data being generated, edge computing at the network level has become an important intermediate layer. It can perform basic real-time analytics closer to the machines, instead of sending the data to the cloud or an on-premise server.
Middleware software companies such as PTC and Telit act as the horizontal plumbing and data delivery platform, to ensure data reaches the system of record. Use cases include device access and provisioning, data ingestion, API management and protocol conversion.
Security companies provide network topologies, to detect malware, threats and attacks.
In this regard, hardware-focused networking companies have an inherent advantage, because the industrial gateway is the natural real estate to host edge compute, middleware and security software agents. Gateways are hubs to ingest data across different sources, and provide a compute environment for software that needs to sit closer to machines.
While these infrastructure companies process high volumes of network data, they do not have capabilities to extract actionable insights on process optimization.
In the near future, I expect OT infrastructure companies to offer more vertical-specific analytics applications further north in the technology stack.
Data historians:
The frequency and large volumes of sensor data are unique to industrial settings. Time-series databases called historians have become de facto standards, for their storage and compression efficiencies over other databases. Examples include OSI Soft, AspenTech and Wonderware – OSI Soft is estimated to be the biggest historian.
Data historians provide visualization tools on install base data, but lack strong predictive capabilities (despite being the source of truth for most time series sensor data). Several startups extract more insights from the same data sets that the historians have access to. This is partly because of better data modeling and preparation techniques, and the ability to enrich models from other data sources such as ERP, MES systems.
Cloud players:
Most OT deployments are on-premise, mainly for security reasons. Cloud platforms such as AWS Greengrass and Azure have taken a top-down approach by pushing analytics capabilities to the edge or through their private cloud deployments.
However, their products are ‘AWS-lite’ or ‘Azure-lite’ versions for IIoT. They lack deep penetration into OT data sources and resident knowledge around connecting data across different protocols. Their analytics works well if someone provides them with OT data that is already cleaned and homogenized.
Going forward, I believe AWS and Azure will need to make inorganic moves for a deeper IIoT analytics solution.
________________________________________
Key go-to-market (GTM) and Business Model Strategies to Win in the IIoT ecosystem:
Based on the industry characteristics highlighted above, I believe the following GTM and business model strategies are critical for startups to win in the IIoT analytics market:
1) Startups need a strong integrated services strategy
Professional services comprise a large share of revenue for IIoT companies (ARC Research estimates services to be 60% of the analytics spend). Even as the market matures, the OT ecosystem will compel bigger vendors to remain services-heavy. Startups will either need large integration teams or build product integrations with system integrator offerings.
The most natural sales motion for vendors is a land-and-expand strategy – start with a pilot at one site, prove out a solution and then expand deployments to multiple sites within a customer account. However, the expand motion is not as scalable, when compared to other ecosystems.
Sales cycles are long in the industrial world – often, 6-8 months for an account + 1-3 month deployment times for each site. Within the same customer account, it is common for a startup to successfully convert a pilot to a license sale in one site and yet start at a new site with a new pilot or a smaller license sale. Winning a broad enterprise-wide license (for 20, 40, 50+ sites at a time) is difficult and startups can get mired in services-heavy projects.
This occurs because of the data challenges highlighted previously, and the fragmentation of the buying center between OT and IT.
Buying center fragmentation: According to 451 Research, only a third of OT respondents reported that they ‘cooperate closely with IT’ on IoT projects. OT and IT buying centers have different budgets, stakeholders and structures. Often, the IT team has the budget to spend as part of a digital transformation mandate, but the OT team is the key influencer in selecting the vendor. It’s never easy selling to large enterprises, let alone navigating the politics of two disparate buying centers.
Long integration and deployment cycles: As discussed earlier in the blog, data integration tends to be manual, less scalable and not easily replicable across sites. Each factory, site, plant is different – the machine types, brand and connectivity protocols vary. Accordingly, services can still be a big component of expansion revenue within a customer account.
Custom application development: As highlighted previously, data modeling requires OT domain expertise. Custom application development teams are critical to handhold, and work with OT customers to contextualize data models. It is foreseeable that as companies build out libraries of models, the dependency on such teams will reduce. For example, a template for a pump or valve can be used similarly across customers. However, the market is yet to reach a level of maturity for a pure ‘out-of-the-box’ contextualization functionality.
2) Startups need to price segment, based on the criticality of assets
Most IIoT analytics companies do not price differentiate between low-value and high-value assets. The pricing model is based on the number of seats for the visualization dashboard, along with a tiered pricing structure for the number of data sources (which is a function of number of sites and machines penetrated).
Startups also need to think about the value of the underlying machines monitored on their platforms. More IoT devices managed, will not necessarily lead to higher or stable gross margins. For example, IoT device and network management platforms in other verticals, are becoming commoditized – the number of IoT devices managed are increasing, but monetization per device is flat or declining.
OT customers do not care about analytics on every machine equally. Machines breakdown in a factory every day – operators cannot stop production for every minor failure. While they generally work to resolve small issues on the side, a less important machine is not top of mind. In this regard, while all OT customers care about a broad analytics product, they are only willing to pay the big bucks for insights on critical machines.
3) Focus on 2 – 3 verticals
Successful IoT applications tend to be vertical-specific. Early evidence suggests that there may not be a true horizontal IIoT analytics winner. Instead, there would be winners within each vertical across manufacturing, energy, mining, chemicals, utilities, and other non-industrial sectors such as HVAC and healthcare. The protocols, data modeling techniques, customer stakeholders and buying centers vary across these verticals.
4) Build out a channel-led sales model from Day 1
Startups will not win this market alone. Selling to OT customers is difficult because of long sales cycles and less scalable expansion motions within accounts. Startups need to leverage the customer footprint of larger OT players. Similarly, bigger companies lack organic IIoT analytics capabilities and need to partner with startups. In this regard, both sides have the opportunity to scale faster synergistically.